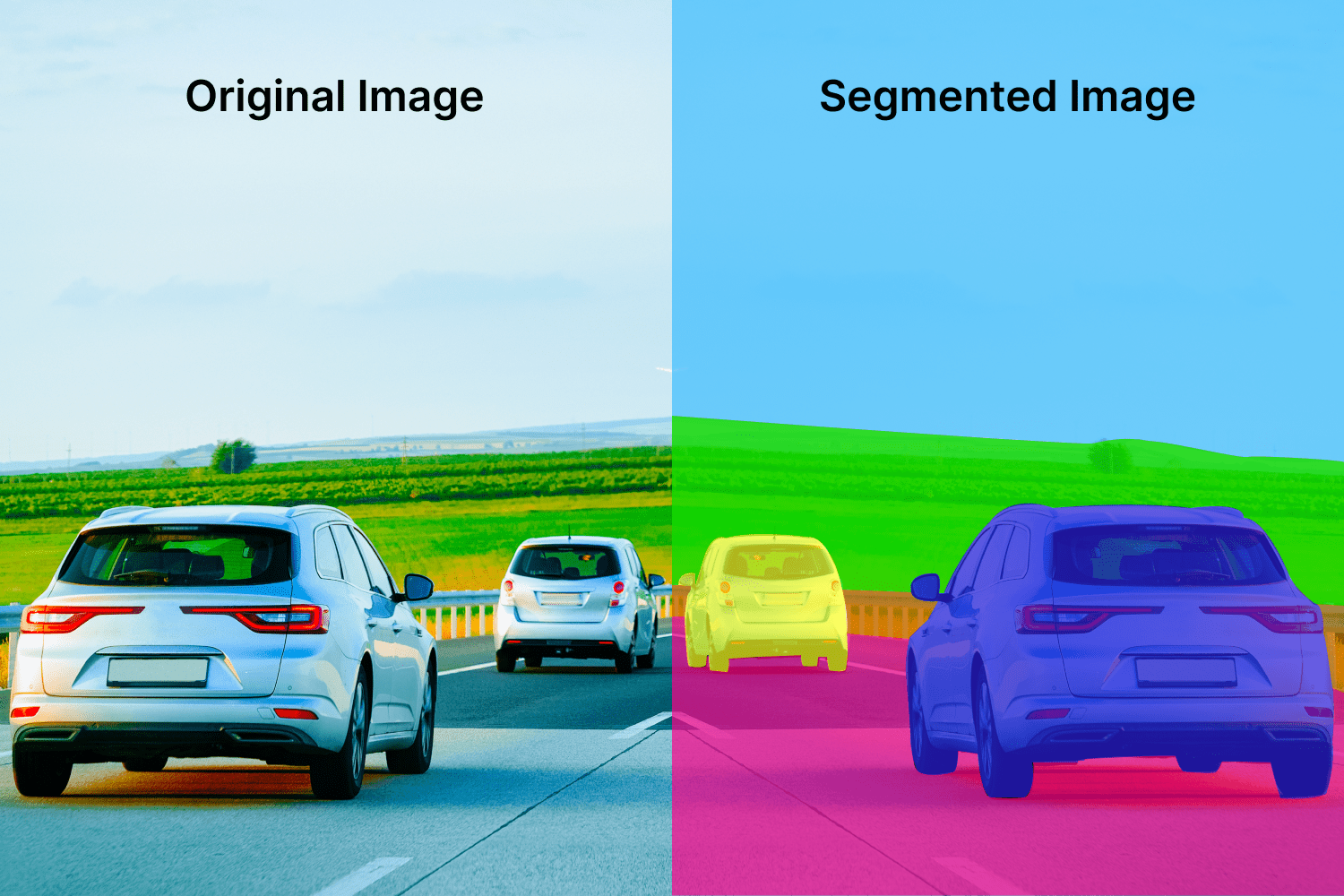
adminMarch 8, 2023Uncategorized
Image segmentation is the process of dividing an image into different parts or segments to identify the objects and boundaries within it. It is one of the most essential tasks in computer vision, as it enables us to extract valuable information from images. Image segmentation has many applications in various fields, including medical imaging, autonomous vehicles, robotics, agriculture, and gaming. In this blog post, we will explore the importance of image segmentation, its applications, and its future.
What are the Benefits of Image Segmentation?
Image segmentation is a powerful tool that enables the identification and isolation of specific objects or areas of interest within an image. One of the primary benefits of image segmentation is the ability to extract relevant information from an image accurately. Dividing an image into smaller, more manageable segments, it makes easier to analyze each segment individually, helping to identify the objects or features within the image. This information is used to make informed decisions or predictions based on the image’s content.
Another significant advantage of image segmentation is the ability to enhance image quality. By isolating the areas of interest, it becomes easier to apply various image processing techniques such as contrast enhancement, noise reduction, and edge detection to effectively remove unwanted elements from an image, such as noise, background clutter, or other unwanted objects.
Additionally, image segmentation plays a vital role in object recognition and tracking. By dividing an image into segments and analyzing each segment’s properties, computer vision algorithms can identify and track specific objects within an image or video stream. This technology has numerous applications in fields such as robotics, where machines can utilize image segmentation to identify and track objects in real time.
What are Some Applications of Image Segmentation?
Image segmentation is one of the assertive techniques of computer vision that has many applications in various fields, including medical imaging, autonomous driving, and video surveillance. Here are a few most important applications that are currently in practice or the stage of advanced research.
Medical imaging
Medical imaging technologies have revolutionized the way doctors diagnose diseases and injuries. Radiography, magnetic resonance imaging (MRI), ultrasound, and computed tomography (CT) are some of the most common medical imaging techniques. However, making sense of the images produced by these machines requires a lot of work. This is where image segmentation comes in.
Tumor detection
Tumor detection is one of the most important applications of image segmentation in medical imaging. With the help of image segmentation, doctors can identify the exact location and size of a tumor. This information is critical for planning the treatment of cancer. Image segmentation works by separating the tumor from the surrounding healthy tissues. This allows doctors to see the tumor more clearly and accurately measure its size. The accuracy of tumor detection using image segmentation is higher than traditional methods.
Brain segmentation
Another important application of image segmentation in medical imaging is brain segmentation. The human brain is a complex structure made up of different tissues, each with its distinct characteristics. Image segmentation can be used to separate the different tissues in the brain, such as gray matter, white matter, and cerebrospinal fluid. This information can be used to diagnose different brain diseases, such as Alzheimer’s disease, stroke, and multiple sclerosis. In addition, brain segmentation can be used to plan surgical procedures and monitor the progression of a disease.
Diagnosis of diseases
Image segmentation for diagnosing a wide range of diseases, including cardiovascular disease, lung disease, and bone disease. For example, in cardiovascular disease, image segmentation is to measure the size and shape of the heart and its chambers. This information can be used to diagnose conditions such as cardiomyopathy and heart failure. In lung disease, image segmentation can be used to measure the volume of the lungs and detect abnormalities such as tumors and nodules. In bone disease, image segmentation can be used to measure bone density and detect fractures.
Autonomous vehicles
Image segmentation is a crucial strategy for the proper functioning of autonomous vehicles. Object detection, lane segmentation, and semantic segmentation are three important types of image segmentation that enable autonomous vehicles to interpret and understand their environment. With accurate image segmentation, autonomous vehicles can navigate safely and efficiently on the road, making them an essential technology for the future of transportation.
Object detection
Object detection is a type of image segmentation that involves identifying and locating objects within an image. This is an essential process for autonomous vehicles, as it enables them to detect and avoid obstacles on the road. These algorithms can recognize a wide range of objects, from pedestrians and cyclists to other vehicles and road signs. With accurate image segmentation, autonomous vehicles can navigate safely and efficiently on the road.
Lane segmentation
Lane segmentation is another important type of image segmentation for autonomous vehicles. This process involves identifying and separating different lanes on the road. Lane segmentation algorithms use a variety of features, including color, texture, and shape, to differentiate between different lanes. With accurate lane segmentation, autonomous vehicles can stay within their designated lanes while driving, which is essential for safety and efficiency. Lane segmentation is also important for navigation, as it enables autonomous vehicles to follow a specific route.
Semantic segmentation
Semantic segmentation is a more advanced type of image segmentation that involves labeling different areas of an image with semantic information. This process enables autonomous vehicles to understand the meaning of different objects and areas in their surroundings. With semantic segmentation, autonomous vehicles can identify and differentiate between different types of objects and areas, including roads, sidewalks, buildings, and vegetation. This information is crucial for autonomous vehicles to navigate effectively and make informed driving decisions.
Robotics
Robots are becoming an increasingly important part of our daily lives. They are being used in various industries such as manufacturing, healthcare, and logistics, among others. For robots to be effective in these industries, they need to be able to perceive their environment accurately. This is where image segmentation, object recognition, and navigation come in.
Object recognition
Object recognition is important in robotics because it allows the robot to understand its environment and interact with it more effectively. Object recognition in coordination with image segmentation can enhance robotics to better understand their environment and follow their commands more precisely. For instance: A robot is assigned a task to identify an object in an unfamiliar environment. It can apply image segmentation techniques to identify the object and it can use this information to make decisions about how to interact with the object.
Navigation
Navigation is the process of moving a robot from one point to another. This process is essential in robotics because it allows the robot to move around its environment and interact with objects. By combining image segmentation and object recognition with navigation, a robot can move around its environment and interact with objects more effectively. As image segmentation techniques engine the navigation decisions and make it easy for robots to make decisions related to path planning, obstacle avoidance, and localization.
Agriculture
Image segmentation is an emerging technology that can help farmers improve their crop management practices, reduce production costs, and increase crop yield, ultimately leading to a more sustainable food supply. With its ability to estimate crop yield and detect weeds, it has the potential to revolutionize the agricultural industry.
Crop yield estimation
Image segmentation can be used to estimate crop yield by analyzing the vegetation index of crops. Vegetation indices are calculated using the spectral reflectance of crops, which can be measured through remote sensing. Image segmentation techniques can then be applied to these images to identify and count crops, making it possible to estimate crop yield accurately.
Weed detection
Weed detection is another crucial application of image segmentation in agriculture. Traditional weed detection methods involve manual labor and are time-consuming. Image segmentation technology can help farmers detect weeds in real-time, allowing them to take timely action to remove them.
Image segmentation techniques can be used to differentiate between crops and weeds based on their spectral reflectance. This can be done by analyzing the color, shape, and texture of the plants with high accuracy.
Gaming
In the gaming industry, image segmentation is used to create realistic and immersive game environments. The technique is used to separate game elements such as characters, objects, and backgrounds. This allows game developers to manipulate each component independently, resulting in a more dynamic and interactive game. Image segmentation is also used to detect and track player movements, which is crucial for games that require motion control.
Virtual reality
Image segmentation is used in VR to create realistic and interactive virtual environments. The technique is used to separate virtual objects from the background, resulting in a more immersive experience for the user. Image segmentation is also used in VR for gesture recognition, which allows users to interact with virtual objects using hand movements.
Augmented reality
Image segmentation is used in AR to separate real-world objects from virtual ones. This allows the virtual objects to interact with the real world seamlessly. Image segmentation is also used in AR for object recognition, which enables the technology to recognize and label real-world objects.
What are the Most Common Techniques for Image Segmentation?
Image segmentation is a crucial task in computer vision and image processing. There are various techniques available for image segmentation.
1. Edge-Based Segmentation
Edge-based segmentation is a popular technique that identifies the edges of objects in an image. This technique uses edge detection algorithms to locate the features of associated objects using the information from the edges. Edge-based segmentation algorithms can accurately represent the borders of objects in an image using edge chains comprising individual edges.
2. Threshold-Based Segmentation
Threshold-based segmentation is a simple technique that segments an image based on the intensity of pixels relative to a given value or threshold. It can accurately segment objects with higher intensity from other objects or backgrounds. Thresholding divides a grayscale image into two segments based on their relationship to the threshold value.
3. Region-Based Segmentation
Region-based segmentation involves dividing an image into regions with similar characteristics. The algorithm locates the seed point and grows the regions by adding more pixels or shrinking and merging them with other points. This technique is useful for segmenting objects with similar textures or colors.
4. Cluster-Based Segmentation
Cluster-based segmentation uses clustering algorithms to group pixels with similar characteristics into clusters. This technique is useful for identifying hidden information in images, such as patterns or structures. The algorithm divides images into clusters of pixels, separating data elements and grouping similar elements into clusters.
5. Watershed Segmentation
It is a technique that treats images like topographic maps, with pixel brightness determining elevation. This technique detects lines forming ridges and basins, marking the areas between the watershed lines. It divides images into multiple regions based on pixel height, grouping pixels with the same gray value. This technique is useful for medical image processing and can help identify differences between lighter and darker regions in an MRI scan, potentially assisting with diagnosis.
The Future of Image Segmentation: What Can We Expect?
Image segmentation has already proven to be a valuable tool in various fields. As technology continues to advance, we can expect to see even more innovative applications of this technique in the future. One of the emerging potential applications of image segmentation is in the field of security. By segmenting images of people in public places, security personnel can quickly identify potential threats and take necessary actions. This can help to prevent crimes and maintain public safety.
As industries of every size and feild are growing interest in computer vision applications and image segmentation is the main pillar that influences most computer vision applications. The growth of image segmentation in the coming years is going to be prompt, predominantly in geospatial data analysis, video surveillance, medical imaging, and satellite image analysis.
At Deeplobe, our team of experts is well knowledged about computer vision applications including image segmentation. Willing to know more about text analytics and computer vision applications? The DeepLobe team can potentially help you integrate and benefit from various image segmentation techniques. Contact us for a free consultation.