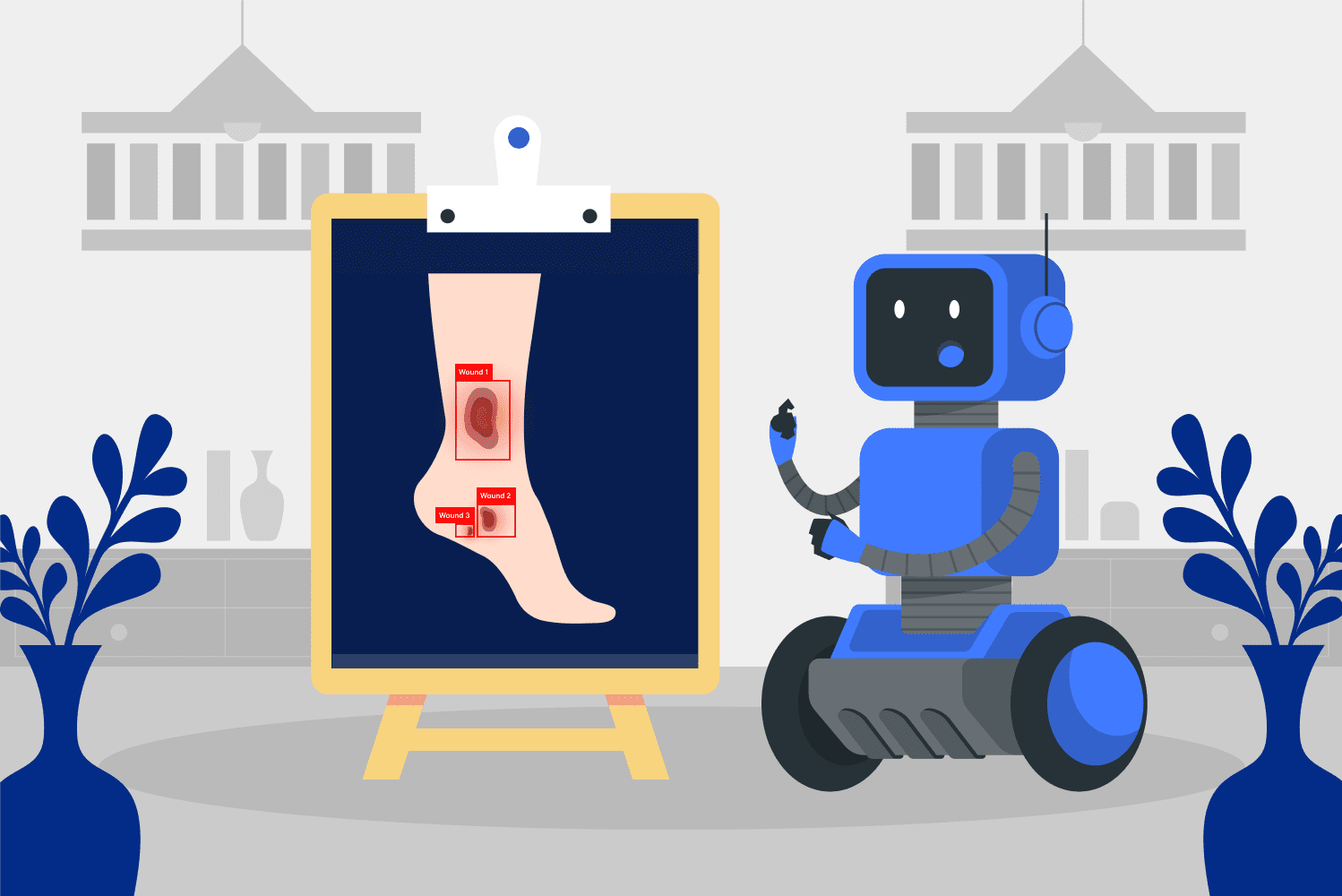
adminJanuary 18, 2024Uncategorized
Diabetes wound detection stands at the forefront of healthcare challenges, demanding innovative solutions for timely intervention. In this pursuit, the integration of artificial intelligence (AI) emerges as a beacon of hope, offering unparalleled precision and efficiency. This blog unravels the transformative landscape of diabetes wound detection, spotlighting the pivotal role AI plays in revolutionizing the identification and classification of these wounds. Our exploration delves deep into the intricacies of AI technologies, unveiling the key features essential for discerning the subtle nuances within diabetes-related wounds.
The Complexity of Diabetes Wound Images: A Visual Puzzle
Diabetes-related wounds present a visual puzzle with unique features that demand precise identification. Understanding the distinctive characteristics, such as color variations, texture changes, and the presence of exudate, forms the foundational step in training AI to recognize and interpret these subtle alterations over time.
The Intricacies of CNNs in Healthcare Imaging
Convolutional Neural Networks (CNNs) have emerged as a powerhouse in image recognition, proving instrumental in processing intricate details within diabetes wound images. Let’s explore how CNNs operate and why they are particularly suited for the nuances of healthcare image analysis.
Within the intricacies of healthcare imaging, the adaptability of CNNs proves instrumental. Specifically tailored for the demands of diabetes wound detection, CNNs excel at deciphering the multifaceted nature of medical images. CNNs leverage convolutional layers to automatically learn relevant features from input images. In the context of diabetes wound detection, these layers become adept at recognizing subtle variations in color, capturing the intricate textures associated with different wound stages, and discerning the presence or absence of exudate – factors crucial for accurate classification.
The technical prowess of CNNs is accentuated by their ability to learn hierarchical representations. As layers progress through the network, higher-level features are extracted, allowing the model to comprehend complex structures within diabetes wound images. This hierarchical learning is especially valuable when dealing with the evolving nature of wounds over time, as CNNs can discern temporal changes in the visual cues presented.
In diabetes wound detection, the spatial hierarchies captured by CNNs play a pivotal role in the accurate identification of wound characteristics. For instance, the network can recognize spatial arrangements of pixels that signify specific wound features, contributing to a nuanced understanding of the image content.
Moreover, CNNs are equipped with pooling layers that downsample the learned features, enhancing computational efficiency without compromising the model’s ability to capture essential details. This downsampling is particularly advantageous when processing high-resolution images, ensuring that the model remains scalable for application in diabetes wound detection scenarios where image quality is paramount.
Unpacking Transfer Learning
Transfer learning operates on the premise that a model pretrained on a large, diverse dataset can transfer its acquired knowledge to a more specific task with a limited dataset – a scenario commonly encountered in medical imaging. In the context of diabetes wound detection, where obtaining extensive labeled datasets is challenging, transfer learning becomes a strategic approach.
The technical underpinnings of transfer learning involve the adaptation of a pretrained model’s weights and parameters to the target task. For diabetes wound detection, this means fine-tuning a model initially trained on a general image dataset to recognize features specific to diabetes-related wounds. This process significantly reduces the need for exhaustive training on a limited medical image dataset, accelerating the deployment of effective models.
One of the key advantages of transfer learning lies in its ability to capture generic features during the initial training phase and subsequently refine them for task-specific nuances. In diabetes wound detection, where distinct visual cues define the characteristics of interest, transfer learning facilitates the extraction of these features with remarkable efficiency.
In the domain of diabetes wound detection, transfer learning becomes a catalyst for achieving high classification accuracy. By harnessing the knowledge embedded in pretrained models, the technology can discern intricate details within medical images, including those specific to diabetes wounds, contributing to a more robust and adaptive AI solution.
Navigating the Algorithmic Landscape: AI Models Tailored for Wound Detection
Let us now navigate the algorithmic landscape, exploring how specific AI models, such as Support Vector Machines (SVMs) and Recurrent Neural Networks (RNNs), play pivotal roles in diabetes wound detection. Understanding their applications and strengths provides a comprehensive view of the diverse approaches to tackling this healthcare challenge.
Support Vector Machines (SVMs) for Classification
Support Vector Machines (SVMs) stand as robust tools in the arsenal of AI models, especially when it comes to classification tasks. At the core of SVMs lies the concept of creating an optimal hyperplane that maximally separates distinct classes within the feature space. In the case of diabetes wound detection, this translates to SVMs efficiently discerning patterns and features that differentiate between healthy tissue and diabetes-related wounds.
The strength of SVMs lies in their ability to handle high-dimensional data, making them well-suited for the complex visual information inherent in medical images. When applied to diabetes wound images, SVMs excel at capturing subtle variations in color, texture, and other visual cues, contributing to their capacity to enhance classification accuracy.
The kernel trick further amplifies SVMs’ capabilities by transforming input features into higher-dimensional spaces, allowing the model to efficiently classify non-linear relationships within diabetes wound images. This becomes particularly relevant when dealing with intricate patterns that might not be discernible in lower-dimensional spaces.
Contrary to deep learning approaches, SVMs offer interpretability, allowing healthcare professionals to comprehend the features contributing to a particular classification decision. This transparency can be crucial in a medical context, providing insights into the visual indicators considered by the model when distinguishing diabetes wounds.
Recurrent Neural Networks (RNNs) for Temporal Analysis
Unpacking the temporal dimension of diabetes wound progression requires a specialized approach, and Recurrent Neural Networks (RNNs) emerge as a formidable solution. Unlike traditional neural networks, RNNs introduce a feedback loop, allowing the model to capture sequential dependencies within time-series data, a crucial aspect in monitoring changes over time in diabetes-related wounds.
RNNs prove invaluable in analyzing the evolution of wounds by considering the temporal sequence of images. This temporal analysis becomes pivotal when tracking changes such as healing progress or the development of complications, enabling a more comprehensive understanding of the dynamic nature of diabetes-related wounds.
The Long Short-Term Memory (LSTM) architecture within RNNs is particularly well-suited for handling sequences with long-range dependencies, a characteristic prevalent in the progression of diabetes wounds. LSTMs maintain a memory cell that can retain information over extended periods, allowing the model to discern subtle temporal patterns and nuances.
Moreover, bidirectional RNNs augment this temporal analysis by processing sequences in both forward and backward directions, capturing a holistic view of the temporal dynamics within diabetes wound images. This bidirectional processing enhances the model’s ability to recognize intricate patterns that might be missed in a unidirectional approach.
Towards a Comprehensive Solution in Diabetes Wound Detection
We look towards the future of diabetes wound detection, acknowledging the continuous evolution of AI in healthcare. Encouraging ongoing research and collaboration between IT companies and healthcare institutions remains pivotal in refining models for improved diabetes wound detection.
The Dynamic Evolution of AI in Healthcare
The trajectory of artificial intelligence within healthcare is dynamic and ever-evolving. Embracing the future of wound detection means acknowledging the continuous refinement of AI technologies. As algorithms become more sophisticated and computing capabilities advance, the potential for even more precise, efficient, and accessible solutions in healthcare imaging expands.
AI’s evolution extends beyond just improved model architectures. It encompasses the integration of emerging technologies, such as edge computing and decentralized AI, making it possible to deploy wound detection systems directly at the point of care. This shift towards decentralized solutions holds the promise of real-time analysis, enabling faster interventions and more personalized patient care.
Catalyzing Progress through Research and Collaboration
Encouraging ongoing research becomes a cornerstone in shaping the future of wound detection. The collaboration between IT companies and healthcare institutions catalyzes the refinement of existing models and the development of innovative approaches. It is through shared expertise, insights, and a commitment to pushing the boundaries that transformative breakthroughs are likely to emerge.
Research initiatives can focus on expanding datasets, encompassing a broader range of demographics and wound presentations. This inclusivity ensures that AI models are trained on diverse data, enhancing their adaptability to the unique characteristics of diabetes-related wounds across different patient profiles.
Collaborations between IT companies and healthcare institutions should extend beyond the development phase to the deployment and continuous improvement of AI models. Feedback loops that incorporate real-world clinical experiences contribute to refining models, making them more effective and relevant in diverse healthcare settings.
Moreover, engaging healthcare professionals in the development and implementation processes ensures that AI solutions align with the practical needs of clinicians. User-centric design, informed by the daily challenges faced in clinical practice, results in AI tools that seamlessly integrate into existing workflows, enhancing their utility in diabetes wound detection.
When it comes to healthcare innovation, DeepLobe’s pretrained models stand as a beacon of hope for transforming wound detection. By unraveling the intricacies of distinctive features and advanced algorithms, we pave the way for early intervention and proactive healthcare management. The intersection of technology and healthcare, exemplified by DeepLobe, holds the promise of minimizing the impact of diabetes-related wounds and ushering in a new era of transformative patient outcomes.
As we navigate this boundless landscape, the journey towards comprehensive healthcare solutions becomes an exciting and dynamic trajectory, fueled by the potential of AI and the collaborative efforts of IT and healthcare professionals. For more information on DeepLobe and to book a free demo, contact us.