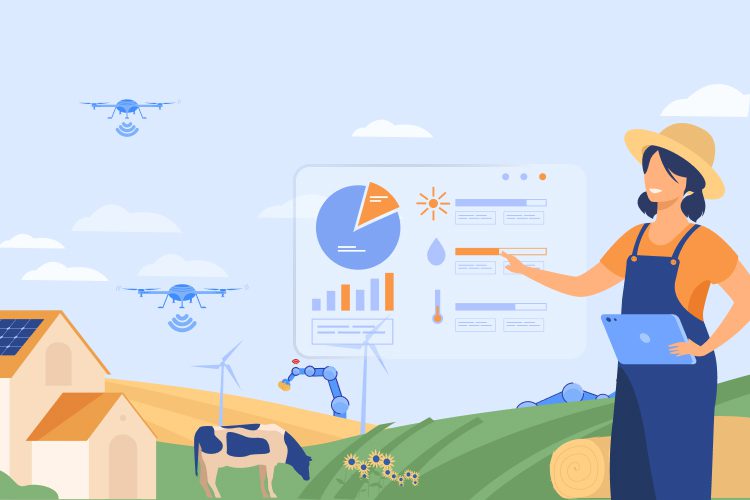
DeepLobeDecember 30, 2020Agriculture , Computer Vision
The recent advancements in Computer Vision technology are upgrading the overview of its applications in agriculture. The combination of Artificial Intelligence, Computer Vision, and Machine Vision is making the world of farming more advanced than humankind ever discovered. Employing Agri-Tech is disrupting the traditional dynamics of farming by helping farmers in better crop yielding. Using the framing robots to plant diagnosis applications, Computer Vision and Deep Learning models bring tremendous change. As the prominence of Computer Vision in agriculture continues to grow, the Zion market research has estimated that this technology can provide a sustainable future for farming.
Technology helps farmers grow healthier crops, control their usage against pesticides, analyze and monitor soil conditions, measure plant growth in real-time, etc. New-age farming or smart agriculture is contributing to the global food life cycle by minimizing the total cost of production and improving the agricultural production outcomes. As the significance of AI in farming is strengthening, it brings in benefits of data-driven predictive decision-making, computer vision-driven inspective farming, etc. The application of state-of-art technologies to monitor, analyze, and enhance the food production chain from photosynthesis to plant growth. In this article, we described the evolution of computer vision applications in agriculture and how these applications are enlightening the field of farming.
Computer Vision & Smart Agri-Tech
With the increasing global population, the challenges related to food are becoming a tremendous concern for any nation. According to the UN reports, this expected population growth by 2050 would require 70% more production than current food production. And it is also important to address the critical issues that are affecting the productivity of the crop. Smart Agriculture is a resolution that meets the current demands and necessities of the food supply around the world. However, employing Agri-Tech is still in the prototyping stage in major countries. And incorporating AI, machine learning, computer vision, deep learning, and information technology, etc., in agriculture promises to scale the future of farming by offering sustainable agriculture practices.
Computer Vision is a machine’s ability to visualize, auto-detect, analyze, and understand the available information from a single image or a sequence of images. The system further guides the user in making reliable decisions with the help of extracted information in real-time. The continuous application of Artificial Intelligence and Computer Vision together widens smart Agri-Tech importance to deliver the right information at the right time to the farmers. With the help of information technology, smart agriculture is becoming more beneficial than ever before. Guiding farmers in remote areas to distant farmlands where the reach of an expert in crop production is impossible, Computer Vision is helping in adopting precision farming techniques.
It dates the history of precision agriculture back to the 1980s. Soil sampling, Geographical Information Systems, yield Mapping, Global Positioning Systems, Automate Cultivation Navigation application, and Robotics are a few precise farming techniques adopted today. Precision farming is one of the top innovations in modern agriculture research powering the growth of AI, Computer Vision, Deep Learning, and Machine Learning practices in agriculture. Smart farming or smart agriculture is employed with advancements in information technology to drive the future of precision farming.
Advancements of Computer Vision Applications in Agriculture
The ability of computer vision applications is transforming agriculture in adopting state-of-art techniques at a speed to improve productivity and reduce the time of production. Here, we have discussed a few tested examples of -how computer vision is transforming modern-day agriculture.
Crop-yield quality and quantity enhancement
Applying computer vision can drive the opportunities to produce a high-quality yield that is rich in minerals, vitamins, and packed with all the beneficiary nutrition for good health. Computer Vision and Machine Learning algorithms are guiding farmers with detailed information about performing certain tests like soil richness, crop growth, natural treatments to pest control, and more. From seed germination to crop harvesting, with the availability right information, precision planning, and sustainable solutions farmers are more focused on enhancing the quantity of yield without compromising on the quality.
Computer Vision tech feasibility to identify the contaminated food products and defects in crop yield by categorizing the total production based on color, shape, size, and surface is optimizing the quality like never.
Plant diseases
Detection of plant diseases at the right stage and the right time benefits the farmers in following the precautionary measures for better crop health. Computer Vision technology can smartly monitor distinct (small, medium, to large-sized) farms seamlessly for better results. The image segmentation and image identification techniques are applied to identify and study an affected plant and the diseases by its symptoms on the plant leaf. More than ever, image identification and image segmentation techniques can study different parts of a plant to detect various diseases using genetic algorithms.
Grading and sorting of fruits and vegetables
Deep Learning-powered object detection models are trained with modern Computer Vision algorithms to classify and grade the food identity based on trained parameters. For instance, a Deep Learning algorithm trained with a set of data containing images of good fruits (let’s consider grapes) and another set of grapes images with the defects -the model by itself identifies and grades the quality of the yield accordingly to deliver more accurate results. Automatic crop grading and sorting of defective harvest are reducing the manual efforts, delivery time, and cost of production at scale.
Phenotyping
Advanced computer vision algorithms are routing the precision and robustness to phenotyping projects to follow precise measurements to study a prototype. During this process, in every farming field, at least a single camera will be there to monitor and observe 1 to 3 plants in a row. The system collects sample images from time to time to analyze and identify the features of the plant – like its height, width along with regions, color, and estimated fruit yield. And by implementing advanced algorithms of deep learning and machine learning to study plant structures (such as flowers and fruits) along with the parameters regarding their dimensions, surface, color, and yield.
Indoor farming
Image processing is a vital practice of precision agriculture that advancing the growth of indoor farming. Monitoring and measuring artificial climate conditions, assessing plant growth, identifying plant diseases, researching soil fertility dynamics, are a few ways how Computer Vision and Machine Learning algorithms are scaling indoor farming.
Computer Vision is one of the prominent reasons for the advancements in smart agriculture or AI-driven agriculture. And the unexplored potential of Computer Vision technology promises to disrupt today’s farming to enhance global food productivity and the global food economy. DeepLobe’s Machine Learning APIs powered with sustainable algorithms can help farmers in employing the next-gen Computer Vision applications to monitor, measure, classify and identify different aspects of farming for better outcomes. For more information or to know how to leverage a machine learning API, connect with us.
Â