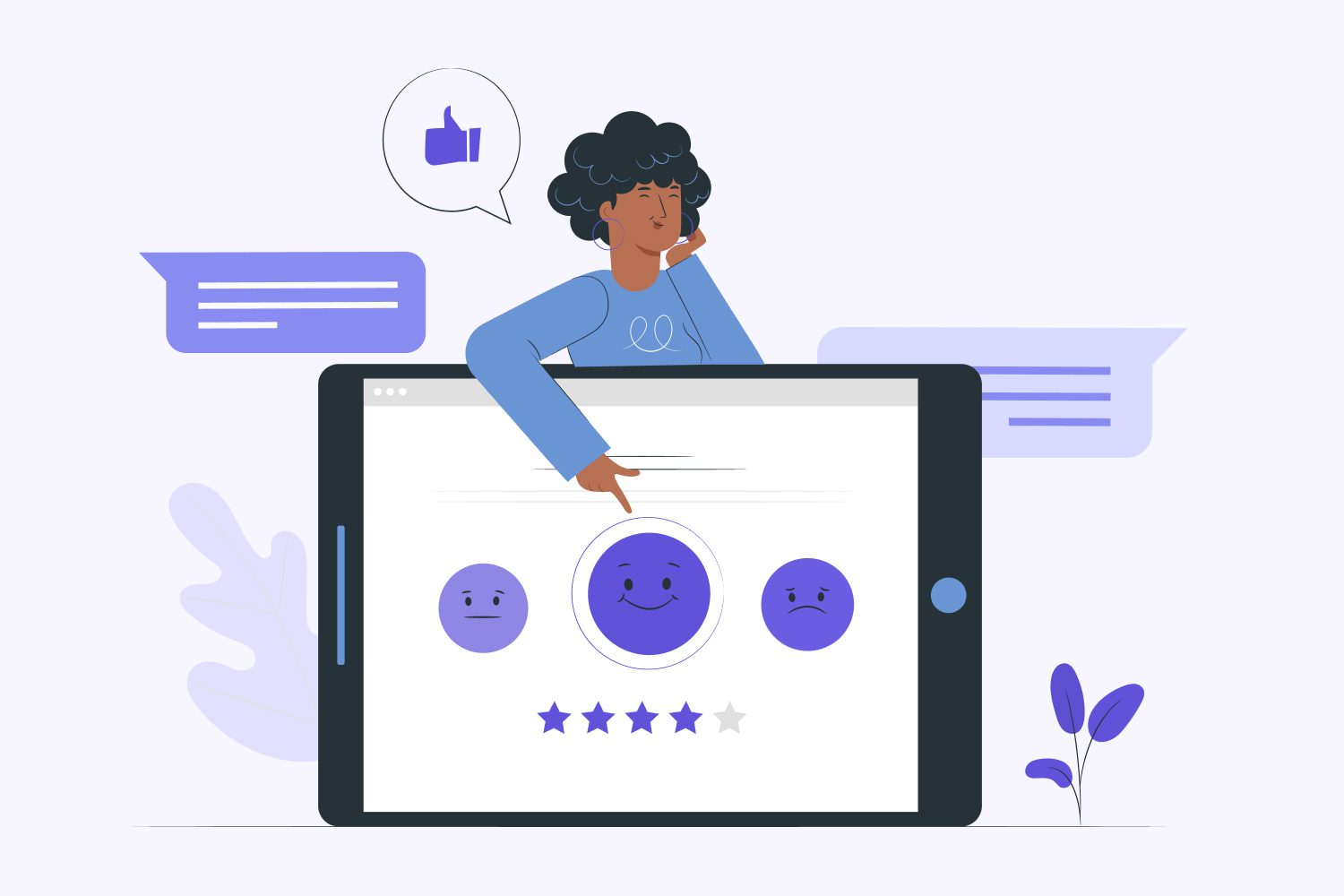
DeepLobeFebruary 11, 2021Deep Learning , Machine Learning , Sentiment Analysis
The increasing demand to channelize customers’ emotions along with their positive and negative reactions for optimizing operations and increasing revenues. Analyzing these reactions and extracting intelligence from them will help brands gauge the ROI of their PR and marketing efforts. And this era of the internet is making it far much easier to collect opinions from people about brands, products, services, or anything. Before the internet, this process of collecting opinions and feedback was done from sources like friends, family, customer reports, etc.
With the advent of social media, it has become much easier to collect diverse opinions from people spread across the globe. There are many sources on the internet to gather opinions on how a particular product or service can be perceived in the market. The eCommerce websites, like Amazon and Flipkart, thrive to provide genuine feedback from their customers in the form of product ratings and reviews, whereas websites like Tripadvisor and Rotten Tomatoes are the places where users can leave their opinions online.
If a particular brand captures all these opinions, by harnessing next-gen technologies, they can analyze the emotional tone behind a particular opinion and offer key insights for customer satisfaction.
Sentiment analysis is the process of identifying and categorizing the opinions expressed within the text – the content on social media, news reports, reviews, etc., – into positive, neutral, and negative. A sentiment analysis architecture uses a combination of Natural Language Processing (NLP) and Machine Learning techniques to allocate the sentiment values to the topics, themes, and categories within the sentences.
Machine Learning For Sentiment Analysis -Overview
Machine Learning improves the accuracy of the low-level text analytics functions at a scale, which sentiment analysis relies on. It helps the data analysts solve the tricky issues arising from the inconsistencies of natural language. We can train the machines with a varied variety of techniques and complex algorithms to perform sentiment analysis.
A logistic regression model trains swiftly on larger datasets and gives robust results. Other models like Support Vector Machines (SVMs), Random Forests, Naive Bayes, etc., can be trained on individual tokens, bigrams, and tri-grams allowing the classifier to pick up negations and short phrases. With the advent of Deep Learning, a new standard to measure sentiment analysis models have been developed. Most advanced models transform the input text into embedded representations, where additional accuracy can be achieved by using pre-trained embeddings such as Word2Vec, GloVe, BERT, or FastText, etc.
A convolutional neural networks model performs convolutions particularly well in the embedded feature space of the words within sentences. LSTMs and RNNs are the recurrent network models that are ideal for working sequential data where they can repeatedly predict the sentiment. High-performance sentiment analysis models like dynamic memory networks, neural semantic encoder, etc., are developed based on another promising approach called Multi-Task Learning (MTL), where a single model is trained jointly across multiple tasks with the goal of achieving ultimate accuracy in as many domains as possible.
Sentiment Analysis -Challenges To Be Addressed
There are a few challenges that sentiment analysis needs to address for it to become the most perfect tool. While customers’ sentiments are influenced by many psychological, geographical, and emotional factors of the customer, it is advisable to go with larger samples of data.
Apart from that, like many NLP techniques, sentiment analysis also needs to cope with the complexities in the language. Automated systems find it difficult in extracting the true sentiment from negations along with understanding the different degrees of sentiments and conflicting sentiments. They cannot differentiate between sarcasm and genuine text whereas the use of abbreviations and emojis in the text also pose interpretation challenges.
Hybrid Sentiment Analysis System – Therefore, many experts prefer opting for a hybrid approach to perform sentiment analysis where Machine Learning is combined with traditional rules to overcome the deficiencies of each approach/model. Rules-based analysis can be treated as an effective way to build a foundation for PoS tagging and sentiment analysis. However, with the unmanageable growth in the rulesets, Machine Learning needs to be brought in to shoulder the load of complex NLP tasks.
Sentiment Analysis – Modernizing Brand And Market Research
Ever since customers are more expressive about their opinions and thoughts than before, sentiment analysis has become an essentially vital tool to monitor and understand customer sentiments. A basic sentiment analysis follows the below process
- Data/text extraction from various sources.
- Running sentiment classifier on each and every text extracted to determine if the sentiment is negative, neutral, or positive.
- Extracting aspect terms that determine the sentiment and determining the polarity of each such aspect identified.
- Producing a final summary by grouping the aspect sentiments together.
Application of Sentiment Analysis for Business
- Sentiment analysis helps improve customer experience and service by tracking and monitoring the key messages from customers’ opinions, thus helping the customer service department to take relevant and swift actions.
- Sentiment analysis provides insightful business intelligence pertaining to customer preferences, strategies to improve service quality, better future product development, reduce customer churn, and improve how the product is presented.
- It helps in making a thorough market analysis by discovering the latest trends and new business opportunities. By observing customers’ opinions on a specific brand and detecting the key messages, new and targeted marketing strategies can be discovered.
- As sentiment analysis captures the moods and impressions of the customers, it surely is a great way to improve sales revenues.
The applications of sentiment analysis are just not limited to social media monitoring, brand monitoring, improving customer support, customer feedback, market research, competitive research, product analysis, workforce analytics, etc.
And if you have decided to implement sentiment analysis in your business to monitor online conversations to improve products and services and maintain reputation, then DeepLobe is your “go-to” place. DeepLobe is a Machine Learning API as a Service platform that will cater to specific needs of customers having their own analytical and visualization solutions that could benefit from accurate sentiments extracted from customers’ data.