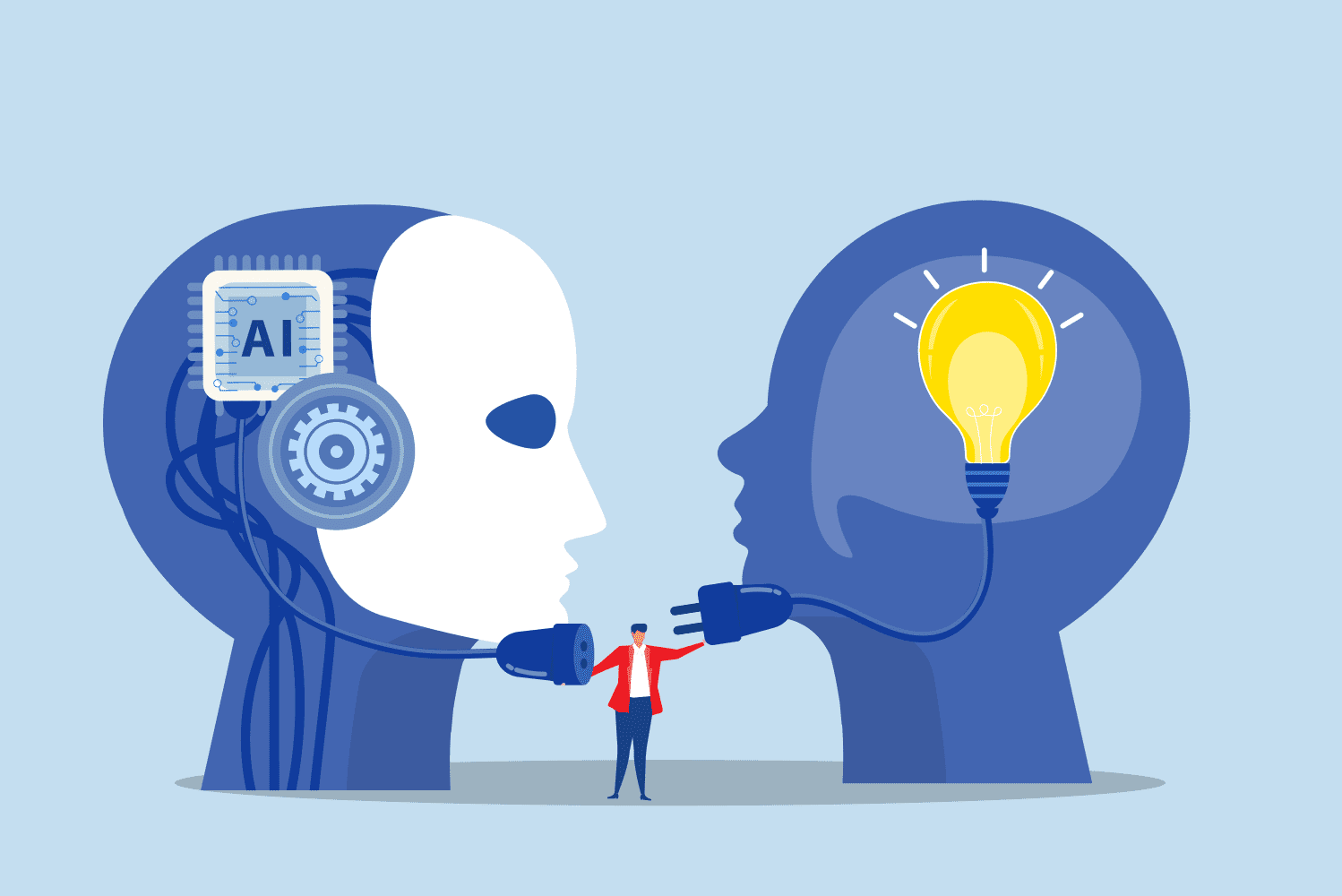
adminAugust 19, 2024Uncategorized
Artificial Intelligence is a technological innovation reshaping industries in ways we couldn’t have imagined. Among the various branches of AI, two that often come up in conversations are Generative AI and Predictive AI. Despite their growing prominence, there’s a lot of confusion around whether these two types of AI are essentially the same or if they serve entirely different purposes. While both offer immense potential and are powerful tools in their own right, they approach problem-solving from distinct angles.
What Is Generative AI?
Generative AI focuses on creating new content, whether it be text, images, music, or even entire virtual worlds. It leverages deep learning models, particularly Generative Adversarial Networks (GANs) and Variational Autoencoders (VAEs), to produce outputs that mimic human-created content.
How Does Generative AI Work?
Generative AI learns patterns from existing data and uses that knowledge to generate new, similar content. For example, a generative AI model trained on thousands of images of cats can create new images of cats that have never been seen before. The model doesn’t just copy the existing data; it understands the underlying patterns and structures to produce new, original content.
Applications of Generative AI
- Content Creation: Generative AI is widely used in content creation, including writing articles, generating art, composing music, and even designing video game levels.
- Design and Creativity: In industries like fashion and architecture, generative AI assists designers by generating new designs based on specific parameters.
- Virtual Worlds and Gaming: In the gaming industry, generative AI is used to create vast, immersive virtual worlds with minimal human intervention.
- Healthcare: Generative AI models can create synthetic medical data that help in training other AI models without compromising patient privacy.
What Is Predictive AI?
Predictive AI focuses on forecasting future events or outcomes based on historical data. It uses machine learning models, such as regression analysis, time series forecasting, and classification algorithms, to predict what might happen next.
How Does Predictive AI Work?
Predictive AI models analyze past data to identify patterns and trends, which are then used to make predictions about future events. For example, a predictive AI model can analyze years of weather data to predict the likelihood of rain on a specific day. The accuracy of these predictions depends on the quality and quantity of the data fed into the model.
Applications of Predictive AI
- Business Forecasting: Companies use predictive AI to forecast sales, market trends, and customer behavior, helping them make informed business decisions.
- Healthcare: In healthcare, predictive AI is used to predict disease outbreaks, patient outcomes, and treatment efficacy.
- Finance: Predictive AI plays a crucial role in financial markets, where it is used to predict stock prices, credit risks, and investment opportunities.
- Supply Chain Management: Predictive AI helps optimize supply chains by forecasting demand, managing inventory levels, and predicting potential disruptions.
Generative AI vs. Predictive AI: Key Differences
Feature | Generative AI | Predictive AI |
Focus | Creating new content | Predicting future outcomes |
Data Usage | Learns patterns to generate new data | Analyzes historical data to identify trends |
Output | Original content | Predictions or forecasts |
Examples | Text generation, image creation, music composition | Fraud detection, customer churn prediction, demand forecasting |
Why the Distinction Matters
- Tailored Solutions
Understanding the distinction between generative and predictive AI is crucial for businesses and professionals looking to implement AI solutions. Knowing which type of AI to use can lead to more tailored and effective solutions, whether it’s for content creation or forecasting future trends. - Avoiding Misapplication
Confusing generative AI with predictive AI can lead to the misapplication of technology, resulting in suboptimal outcomes. For instance, using a generative AI model for a task that requires predictive capabilities could lead to inaccurate results and wasted resources. - Future of AI Integration
As AI continues to integrate into various aspects of life and work, understanding the differences between these AI types will become increasingly important. This knowledge will enable better decision-making and more strategic use of AI technologies.
When to Use Generative AI or Predictive AI
Choosing between generative AI and predictive AI depends on the specific problem you want to solve. Consider the following factors:
- Desired outcome: If you need to create new content or generate ideas, generative AI is the appropriate choice. If you want to predict future events or trends, predictive AI is more suitable.
- Available data: Generative AI requires a large amount of diverse data to learn patterns, while predictive AI relies on historical data with clear patterns.
- Computational resources: Generative AI models can be computationally intensive, while predictive AI models often require less computational power.
While generative AI and predictive AI are distinct concepts, there is some overlap between the two. For example, generative models can be used to create synthetic data for training predictive models. Additionally, predictive models can be used to generate potential scenarios for decision-making.
By distinguishing between generative and predictive AI, we can ensure that these technologies are applied correctly, leading to more innovative and effective solutions. As AI continues to evolve, staying informed about these distinctions will be key to leveraging AI’s capabilities to their fullest.
Contact us for more information on how DeepLobe and Generative AI can help you with your AI projects.