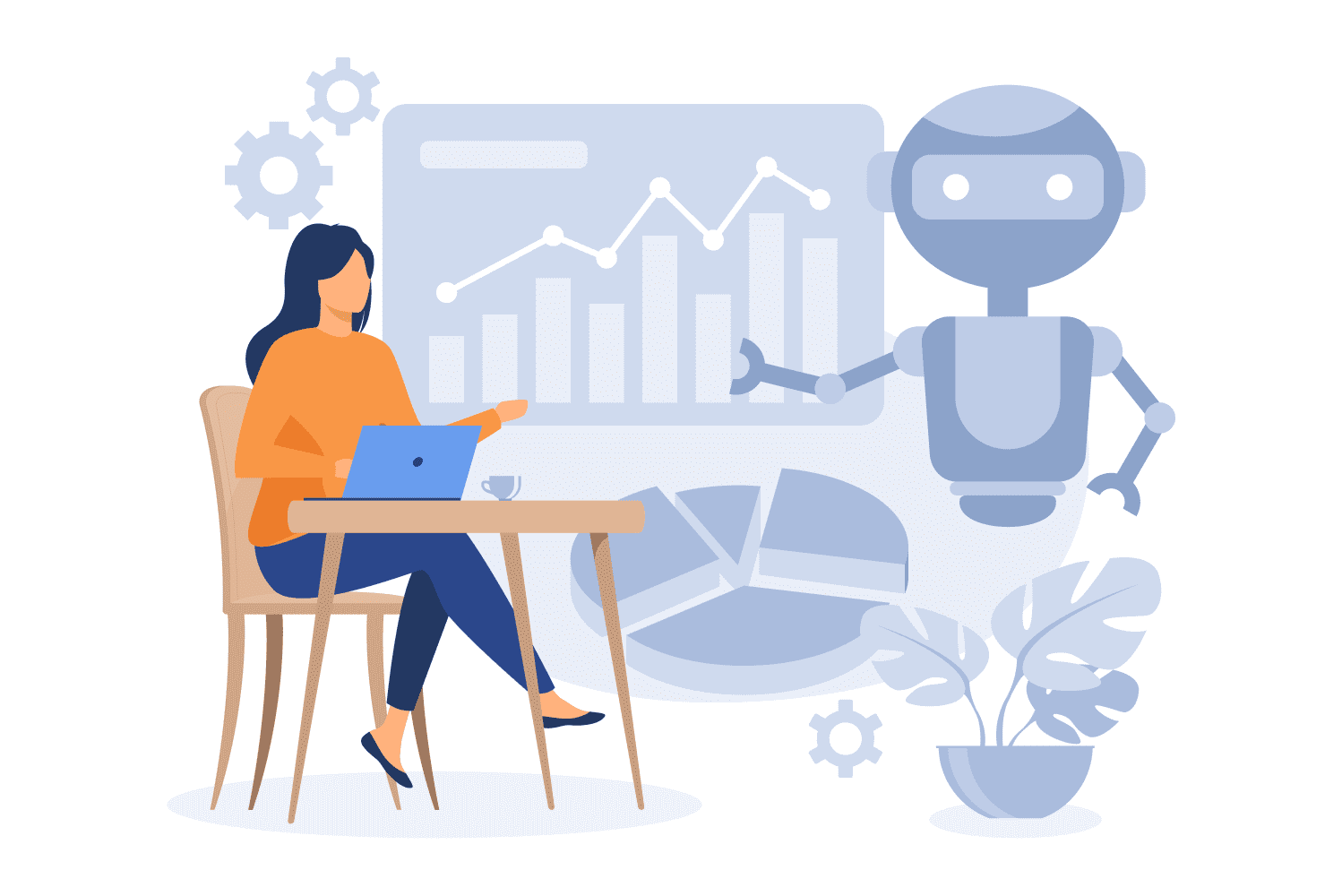
adminJuly 11, 2024Uncategorized
Artificial intelligence is experiencing a transformation with the emergence of Generative AI. This innovative technology can create entirely new content, from realistic images and music to human-like text. It’s revolutionizing industries and changing how we interact with technology and our environment.
Introduction to Generative AI
Generative AI is a branch of artificial intelligence focused on creating new data that mirrors existing data. Unlike traditional AI, which typically categorizes or predicts outcomes based on input data, Generative AI models generate new content. These models can produce anything from synthetic images and voices to entire virtual worlds and stories, learning patterns from extensive datasets to create new, coherent outputs.
The magic behind Generative AI lies in its underlying architectures, such as Generative Adversarial Networks (GANs) and Variational Autoencoders (VAEs).
Generative Adversarial Networks (GANs)
Generative Adversarial Networks, commonly known as GANs, involve two neural networks working in tandem—the generator and the discriminator. The generator creates new data samples, while the discriminator evaluates their authenticity, distinguishing between real and generated data. This back-and-forth process helps the generator improve over time, leading to highly realistic outputs.
Variational Autoencoders (VAEs)
VAEs are another type of generative model. They encode input data into a compressed format and then decode it to recreate the data. This method allows the model to generate new data points by sampling from the compressed representation, ensuring the generated data is similar to the original dataset.
Applications of Generative AI
The applications of Generative AI are vast and varied, touching almost every industry imaginable. Here are a few standout examples:
Content Creation
Generative AI is making significant strides in the creative arts by composing music, creating artwork, and even writing poetry. AI-generated content can assist artists by providing new ideas or fully realized pieces that inspire further creativity.
Healthcare
Generative AI is used to design new drugs and personalize treatment plans. By generating models of new molecular structures, AI can accelerate the drug discovery process, potentially leading to groundbreaking treatments.
Finance
The finance industry benefits from Generative AI through applications in fraud detection and risk management. AI models can simulate various market scenarios, helping institutions better prepare for potential financial outcomes.
Gaming and Entertainment
Generative AI is revolutionizing the gaming industry by creating realistic characters, immersive environments, and complex storylines. This technology enables developers to build expansive worlds that were previously unimaginable.
Marketing and Customer Experience
Marketers use Generative AI to create personalized content at scale, enhancing customer engagement and satisfaction. AI-driven personalization helps deliver targeted advertisements, customized emails, and product recommendations.
The Future of Generative AI
The future of Generative AI holds immense promise. As models become more sophisticated and datasets grow larger, the capabilities of Generative AI will continue to expand. We can expect more seamless integration of AI in our daily lives, from virtual personal assistants who understand and anticipate our needs to AI-generated content that enhances our entertainment experiences.
However, with great power comes great responsibility. The ethical implications of Generative AI cannot be overlooked. Issues such as data privacy, copyright infringement, and the potential for misuse of AI-generated content must be carefully navigated. As we move forward, it is crucial to establish guidelines and regulations that ensure the responsible development and deployment of Generative AI technologies.
Technical Insights: Building Generative AI Models
For those interested in the technical aspects, building a Generative AI model involves several key steps:
- Data Collection and Preprocessing
High-quality, diverse datasets are essential for training effective Generative AI models. Data must be cleaned, annotated, and normalized to ensure the model learns accurately.
- Choosing the Right Architecture
Depending on the application, one might choose GANs, VAEs, or other architectures. Each has its strengths and trade-offs, which must be considered based on the specific use case.
- Training and Optimization
Training Generative AI models requires significant computational resources and fine-tuning. Techniques like transfer learning and reinforcement learning can enhance the model’s performance.
- Evaluation and Refinement
Continuous evaluation using metrics such as FID (Fréchet Inception Distance) and human feedback helps refine the model, ensuring the generated data meets desired standards.
Generative AI is not just a technological advancement; it’s a paradigm shift that has the potential to reshape industries and redefine creativity. From healthcare to entertainment, the applications are as diverse as they are impactful. As we continue to explore and harness the power of Generative AI, it’s essential to remain mindful of ethical considerations and strive for responsible innovation.
At DeepLobe, we are committed to advancing the field of Generative AI, pushing the boundaries of what’s possible, and ensuring that our innovations serve to enhance and enrich human experiences. Join us as we explore the limitless potential of Generative AI and shape the future of technology.